Imagine a world where Artificial Intelligence (AI) can create new content, generate realistic images, and even compose music, all based on patterns learned from data. This is the world of generative AI, a technology that has the potential to revolutionize industries, enhance user experiences, and create new possibilities for human creativity. As we embark on this journey to explore the capabilities of generative AI, prepare to delve into its core concepts, models, tools, and real-world applications while learning about the ethical implications and future predictions of this groundbreaking technology.
Generative AI is no longer just a concept reserved for science fiction; it is becoming an integral part of our daily lives, shaping the way we interact with technology and the world around us. From customer support systems to software development, generative AI has the potential to streamline processes, improve efficiency, and generate valuable insights across a wide range of industries. But how does it work, and what are the key models and techniques that drive its power? Let’s unravel the world of generative AI together.
Short summary
- Generative AI is a powerful technology for creating new content from patterns learned in data.
- It has various models and techniques, with applications across multiple industries.
- Best practices such as emphasizing transparency and monitoring biases should be adopted to ensure responsible use of generative AI.
Unraveling generative AI: core concepts
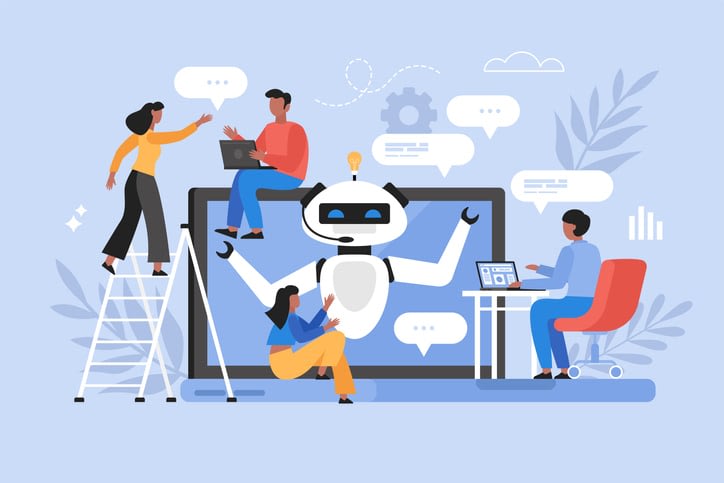
Generative AI, a subset of artificial intelligence, is a powerful technology that utilizes patterns learned from data, such as large language models, to generate new content. Its impact can be seen across various industries and use cases, attracting interest from businesses, professionals, and consumers alike. The roots of generative AI can be traced back to the 1960s, with the creation of the Eliza chatbot by Joseph Weizenbaum, which laid the foundation for modern language models. Since then, advancements in neural networks and deep learning have paved the way for a plethora of generative models, including transformer-based models, Generative Adversarial Networks (GANs), and Variational Autoencoders (VAEs). One such example is the generative AI model, which has significantly contributed to the development of these technologies and the generative AI work in the field, alongside other generative AI models.
The key to generative AI’s ability lies in the training data. A wealth of information is provided to the AI models, enabling them to recognize and replicate various components of datasets, such as:
- Plot structures
- Characters
- Themes
- Narrative devices
The training process, which is essential for developing foundation models in generative AI, benefits from machine learning techniques. These models are then utilized in a myriad of applications, ranging from producing content in response to natural language requests to enterprise scenarios like drug and chip design and material science development.
Key generative AI models and techniques
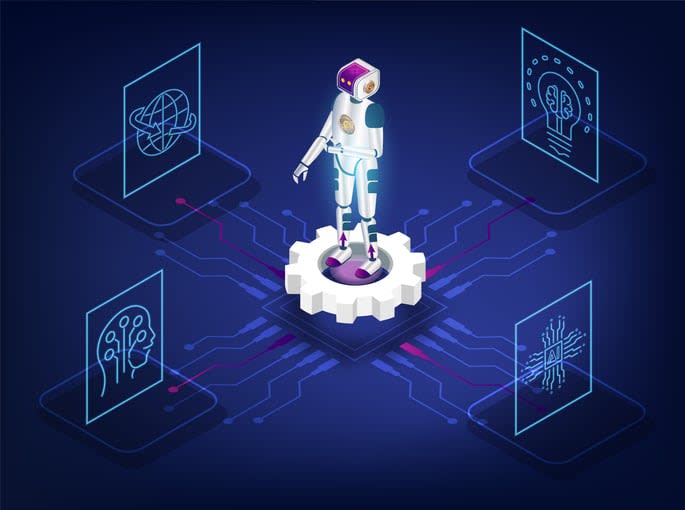
As we delve deeper into the world of generative AI, it’s crucial to understand the key models and techniques that form the backbone of various generative AI systems. These include:
- Transformer-based models
- GANs (Generative Adversarial Networks)
- VAEs (Variational Autoencoders)
- Multimodal models
Each of these models has unique strengths and applications.
Let’s explore each of these models in detail to better comprehend their capabilities and the potential they hold for revolutionizing industries and use cases.
Transformer-based models
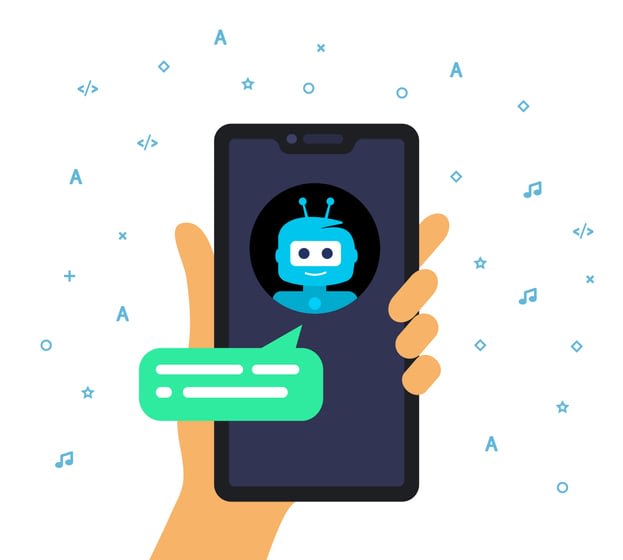
Transformer-based models are a class of AI models that excel in natural language processing and text generation tasks due to their ability to understand relationships between sequential data. These models have been trained on large datasets to comprehend the structure and context of language, making them particularly proficient in tasks such as machine translation and question answering. The primary advantage of transformer-based models lies in their design to process sequential input data non-sequentially, enabling them to more accurately interpret relationships between sequential data and making them suitable for text-generation tasks.
Examples of recent advancements in the field of transformers include Google’s Bidirectional Encoder Representations from Transformers (BERT), OpenAI’s GPT, and Google AlphaFold, which have enabled neural networks to encode language, images, and proteins, as well as generate new content. These state-of-the-art transformer-based models showcase the power of generative AI in revolutionizing the way we interact with language and information, creating new possibilities for applications across various industries.
Generative adversarial networks
Generative Adversarial Networks (GANs) are a deep learning technique introduced by Ian Goodfellow in 2014 that involves the coordination of two neural networks, including recurrent neural networks, to generate and evaluate content variations, leading to highly realistic outputs such as:
- people
- voices
- music
- text
GANs generate realistic outputs through the collaborative efforts of a generator and discriminator, resulting in the creation of authentic-looking data. The discriminator assesses the validity of the generated image, while the generator works to create content that is indistinguishable from real data.
GANs have demonstrated their prowess in generating realistic human faces, making them an ideal choice for tasks that require the creation of authentic-looking data. Their ability to generate realistic outputs has opened up numerous possibilities in industries such as entertainment, advertising, and even security, showcasing the potential of generative AI to impact various aspects of our lives.
Variational autoencoders
Variational Autoencoders (VAEs) are another generative AI technique that uses an encoder and decoder to generate new data by compressing and reconstructing original data, making them suitable for tasks such as generating human faces from photos. The encoder takes the input data and encodes it into a latent representation, which is then passed through the decoder to reconstruct a new representation that is similar to the original data but not identical.
VAEs have proven to be particularly advantageous for tasks that require the generation of realistic human faces using photos as training data. Their ability to compress and reconstruct original data through the use of an encoder and decoder makes them an ideal candidate for applications in industries such as entertainment, advertising, and even medical imaging, further highlighting the potential of generative AI to transform various sectors.
Multimodal models
Multimodal models are a class of generative AI models capable of comprehending and processing multiple types of data concurrently, such as text, images, and audio, enabling them to generate more complex outputs. These models can process multiple types of data, resulting in sophisticated outputs such as images generated from text prompts, showcasing their versatility and potential for a wide range of applications. As a large language model, they also excel in understanding and generating human-like text responses.
Examples of multimodal models in generative AI include DALL-E 2 and OpenAI’s GPT-4, which are used to generate content that seamlessly integrates various forms of data, such as text and images, or even audio and video.
Popular generative AI Tools: ChatGPT, DALL-E, and Google Bard
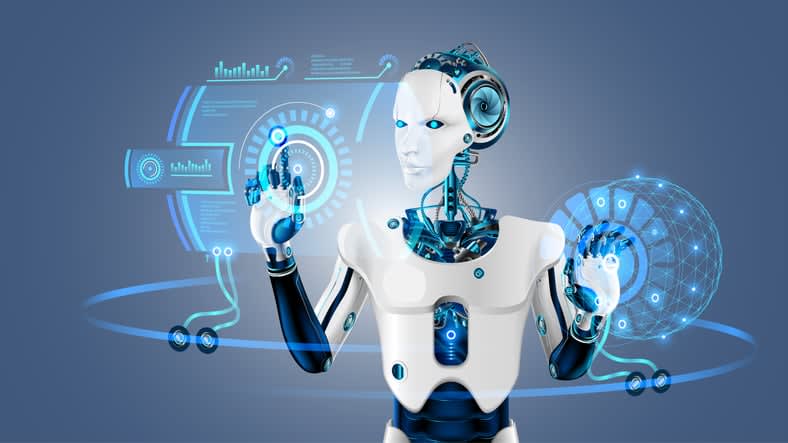
Generative AI is not limited to theoretical concepts and research; it has taken shape in the form of popular tools and applications that showcase its potential impact on various aspects of our lives. ChatGPT, DALL-E, and Google Bard are prime examples of generative AI tools that have made significant strides in improving user experience and providing valuable solutions across different industries.
ChatGPT, associated with Microsoft, is an AI-powered chatbot utilizing OpenAI’s GPT-3.5 implementation that demonstrates the potential of generative AI in creating human-like responses and enhancing customer support systems. DALL-E, a multimodal AI application, recognizes relationships between multiple media such as vision, text, and audio, connecting words to visual elements, paving the way for innovative content creation and design.
Google Bard, a generative AI chatbot, showcases the potential of AI-generated content in transforming how we interact with information and communicate with each other. These tools exemplify the versatility and potential of generative AI across a wide range of applications and industries.
Advantages of implementing generative AI
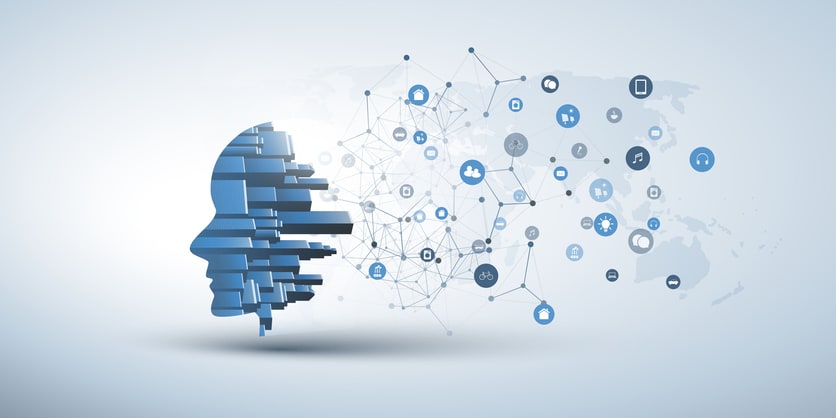
The benefits of generative AI are far-reaching, with the potential to transform industries and use cases by automating or augmenting processes, increasing efficiency, reducing labor costs, and improving operations. For instance, generative AI tools can enhance customer experience and retention, revenue growth, cost optimization, and business continuity. Furthermore, generative AI can facilitate businesses in expediting product creation, accessing new revenue streams, and ensuring compliance with company-specific best practices for writing and formatting code, making it more readable and consistent.
Generative AI can be highly advantageous to professionals and content creators in various areas related to content creation. Its uses range from idea generation, content planning and scheduling, SEO, marketing, engagement with audience, research, and even editing. Additionally, these tools can be used in software development to enhance chatbot performance, classification of content for specific use cases, question answering and discovery, simplification, software coding, summarization, text manipulation, and written content augmentation and creation.
Despite these advantages, it is important to note that the implementation of generative AI comes with its own set of challenges and risks, including the potential for misinformation, biases, and job displacement. As such, it is crucial for businesses and organizations to carefully consider the ethical implications and potential consequences when implementing generative AI in their operations.
Real-world applications of generative AI
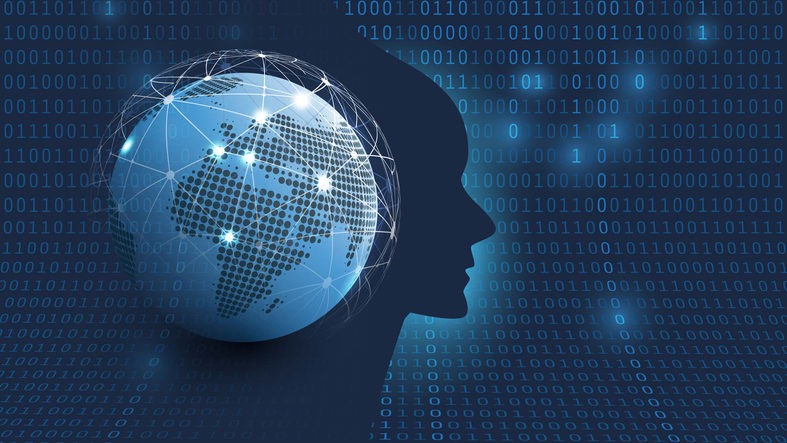
Real-world applications of generative AI span across different industries, demonstrating its practicality and potential impact on various aspects of our lives. Generative AI is playing an instrumental role in the development of transportation, natural sciences, and entertainment. In business, teams can leverage generative AI models to effectively process and categorize third-party data for enhanced risk assessments and opportunity analysis. Generative AI has a potential to be used in semantic web applications. It can be applied to mapping internal job skills taxonomies to various taxonomies used on training and recruitment sites.
Generative AI models have proven their worth in various applications, such as:
- Chatbot performance improvement
- Classification of content for specific use cases
- Question answering and discovery
- Simplification
- Software coding
- Summarization
- Text manipulation
- Written content augmentation and creation
These real-world applications showcase the versatility and potential of generative AI in transforming industries, improving efficiency, and reducing workloads, further emphasizing the need for continued advancements and responsible implementation of this technology.
Challenges and risks associated with generative AI
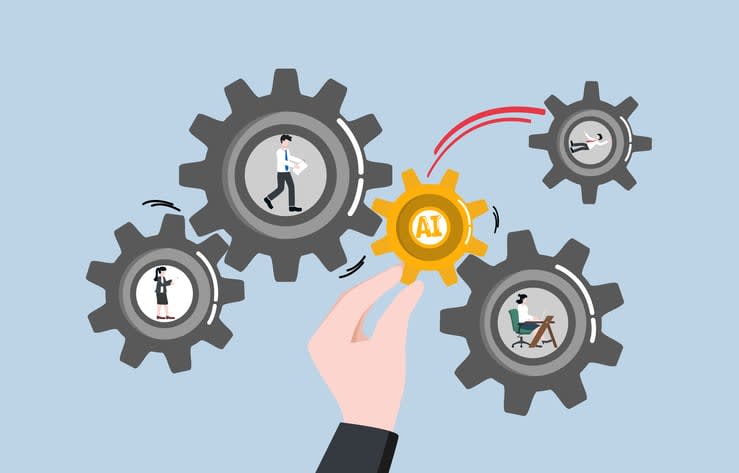
While the potential of generative AI is immense, it is important to recognize and address the challenges and risks associated with its implementation. Some of the concerns include:
- The potential for generative AI to disseminate misinformation
- The perpetuation of biases in AI-generated content
- The displacement of jobs due to automation
- The difficulty of detecting AI-generated content and recognizing when something is incorrect
- Ethical considerations such as accuracy, trustworthiness, bias, plagiarism, and the possibility of misuse or abuse
These challenges and risks must be taken into account when utilizing generative AI.
To mitigate these risks, it is essential to:
- Prioritize transparency
- Conduct internal testing prior to external use
- Monitor biases
- Safeguard privacy when employing generative AI
By addressing these challenges and risks, businesses and organizations can harness the power of generative AI responsibly and ethically, ensuring its potential is fully realized while minimizing negative consequences.
The ethical landscape of generative AI
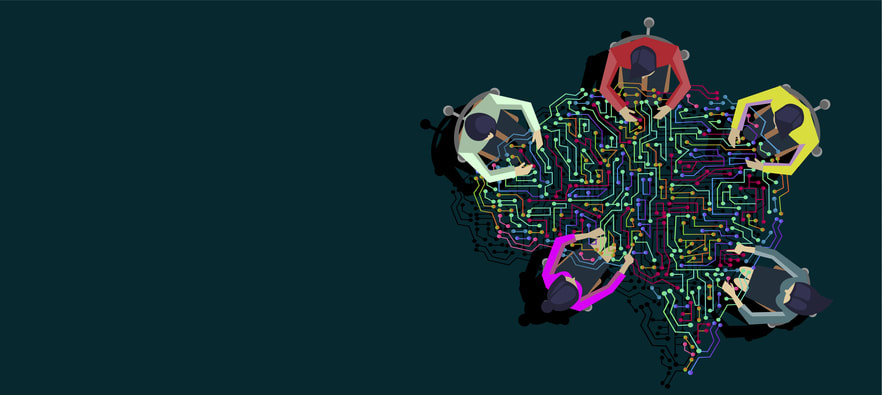
The ethical landscape of generative AI is a complex and evolving area, with concerns surrounding:
- Accuracy
- Trustworthiness
- Bias
- Plagiarism
- Potential for misuse or abuse of the technology
Ensuring accuracy in generative AI is crucial, as the capability of the model to generate outputs that are near the expected outputs directly affects the reliability and trustworthiness of the AI. In addition, monitoring biases is essential to identify potential problems with AI and guarantee that it is operating correctly.
Safeguarding privacy is of utmost importance when employing generative AI, as it assists in guaranteeing that the AI is not collecting or utilizing any personal data without the user’s authorization. By addressing these ethical concerns and implementing responsible practices, businesses and organizations can ensure that generative AI is used in a manner that benefits society while minimizing potential harm.
Generative AI in the business world
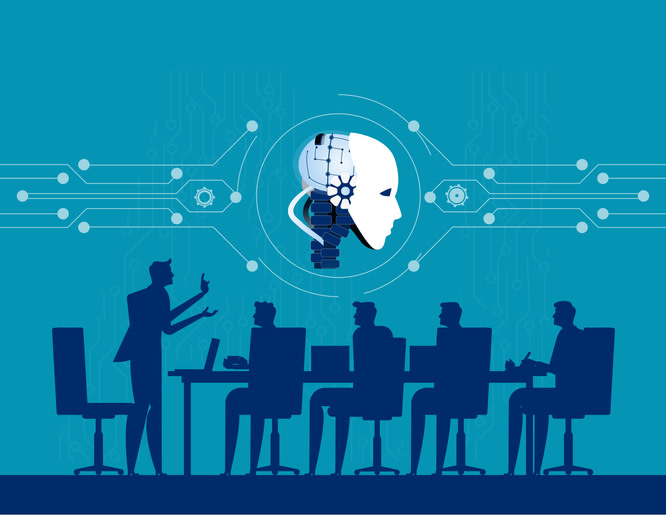
In the business world, generative AI can provide a competitive advantage by automating or augmenting processes, improving customer experience, and optimizing costs. Companies like Kumospace, Google, Microsoft, Amazon Web Services (AWS), IBM, Salesforce, and SAP are major players in the generative AI market, showcasing the potential impact of this technology on various industries and sectors. By leveraging generative AI, businesses can enhance customer experience and retention, revenue growth, cost optimization, and business continuity, paving the way for increased efficiency and innovation.
Generative AI holds the potential to transform the way software development teams work by:
- Ensuring compliance with company-specific best practices for writing and formatting code, making it more readable and consistent
- Facilitating businesses in expediting product creation
- Accessing new revenue streams
- Uncovering valuable insights from enterprise data
By harnessing the power of generative AI and implementing it responsibly, businesses can unlock new opportunities and drive growth in an increasingly competitive landscape.
Best practices for utilizing generative AI
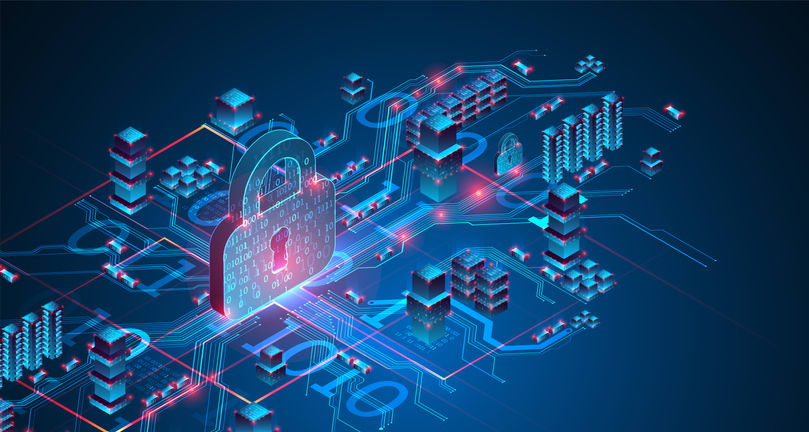
When utilizing generative AI, it is crucial to adopt best practices to ensure responsible and ethical use. Emphasizing transparency is essential, as it aids in guaranteeing that the AI is being utilized responsibly and ethically. Prior to external use, internal testing is of paramount importance when leveraging generative AI to guarantee that the AI is operating correctly and is not introducing any unforeseen biases or errors.
Monitoring biases is essential when utilizing generative AI to identify any potential problems with the AI and guarantee that it is operating correctly. Securing privacy is of utmost importance when employing generative AI, as it assists in guaranteeing that the AI is not collecting or utilizing any personal data without the user’s authorization.
By following these best practices, businesses and organizations can harness the power of generative AI responsibly, ensuring that its potential is fully realized while minimizing potential risks and ethical concerns.
The future of generative AI: predictions and possibilities
The future of generative AI holds significant potential, but its impact depends on ethical use, regulation, and continued advancements in AI development platforms and tools. According to Gartner, it is projected that by 2024, 40% of enterprise applications will have embedded conversational AI, a significant increase from less than 5% in 2020. This trend is expected to continue, with the widespread adoption of AI-augmented development and testing strategies, automation of design efforts for new websites and mobile apps, and engagement of robocolleagues by millions of humans by the year 2026.
Generative AI has a wide range of applications in the future. Industries such as:
- 3D modeling
- Product design
- Drug development
- Digital twins
- Supply chains
- Business processes
The development of machine intelligence, designed to complement human intelligence, stands to benefit significantly from its use. However, the progression of machine intelligence to assist humans in resolving complex issues will require governance, updated regulation, and involvement from a broad spectrum of society.
As we look towards the future of generative AI, it is clear that this technology holds immense potential for transforming industries, enhancing human creativity, and solving complex problems. However, its success will depend on responsible utilization, appropriate regulation, and further progress in AI development platforms and tools. By embracing the power of generative AI and addressing the challenges and risks associated with its implementation, we can unlock new possibilities and drive innovation in an increasingly interconnected world.
Summary
In conclusion, generative AI is a powerful technology with the potential to revolutionize industries, enhance user experiences, and create new possibilities for human creativity. By understanding its core concepts, models, tools, and real-world applications, we can better appreciate the capabilities and potential impact of generative AI on various aspects of our lives. As we continue to explore the world of generative AI, it is crucial to address the challenges and risks associated with its implementation, including the potential for spreading misinformation, perpetuating biases, and displacing jobs.
The future of generative AI is filled with promise, but its ultimate success depends on ethical use, regulation, and continued advancements in AI development platforms and tools. By embracing the power of generative AI and implementing it responsibly, we can unlock new opportunities, drive growth, and transform industries in an increasingly competitive landscape. With a focus on transparency, testing, tracking biases, and protecting privacy, businesses and organizations can harness the power of generative AI to create a more innovative, efficient, and interconnected world.
FAQs
Generative AI models can take various inputs such as text, image, audio, video and code and generate content into any of those modalities. Examples include ChatGPT, DALL-E and MusicLM which are used to generate text, images and music respectively from user-given prompts or dialogue.
These models are becoming increasingly popular in creative industries, as they can be used to generate content quickly and efficiently. They can also be used to create content that would otherwise be difficult or impossible to create manually. This makes them a valuable resource.
Generative AI is a type of artificial intelligence technology that utilizes deep-learning models to generate text, images, code or other types of content from a user prompt. These models have been used to create audio, code, images, text, simulations, and videos with the potential to drastically change content creation.
Generative AI has the potential to revolutionize the way content is created. It can be used to create content quickly and efficiently, with minimal effort from the user. It can also be used to create content that is more creative and unique than what could be created.
Generative AI creates new data based on existing data, while predictive AI uses data to try and determine the future or prevent bad outcomes. Traditional AI is used for analyzing data and making predictions, but generative AI can take the process one step further.
Google DeepMind’s generative AI capabilities are based on powerful algorithms and neural networks that enable it to learn from vast amounts of data and generate creative outputs. This includes the use of large language models (LLMs) which can predict the next word in a sentence.
The Generative AI App Builder is a tool that allows users to quickly build apps connected to their data.
Generative AI refers to deep-learning models that can generate new text, images, video, audio, code, or synthetic data based on the patterns and structure of their training data.
With its recent breakthroughs, this type of artificial intelligence has the potential to revolutionize content creation.