At the intersection of analytics and strategic decision-making lies a constant: the unwavering importance of data in analytics. Data illuminates paths toward growth and innovation, grounding decisions in solid evidence rather than guesswork. This article tackles why the importance of high-quality data in analytics is essential and how it is a game-changer for business. Prepare to dive into the importance of data analytics accuracy, and the edge it provides in the competitive landscape.
Key takeaways
- Data analytics is fundamental for making informed business decisions, with quality data serving as the fuel of digital business decision-making processes.
- High-quality data is essential for precision and reliability in analytics.
- The data analytics process, encompassing collection, processing, analysis, and leveraging predictive analytics, is crucial for drawing valid insights, forecasting trends, and supporting strategic decision-making in various industries.
- Efficient data-driven decision-making, supported by appropriate tools and KPIs, can optimize operational performance, inform marketing strategies, and boost overall business efficacy, as evidenced by practical case studies.
The bedrock of analytics: understanding the role of data
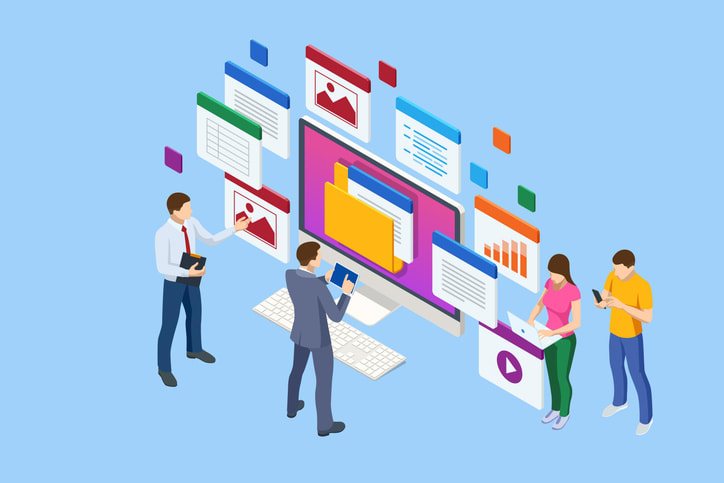
Data, serves as the foundational element in data analytics, crucial for drawing meaningful insights and informing business decisions. From identifying efficient (or weaknesses in) operations to uncovering strategic opportunities, data analytics empowers companies to make informed business decisions. It’s the science of analyzing raw data to extract actionable information.
The ability to recognize trends and test hypotheses based on these insights is a game-changer, transforming the way organizations make evidence-based choices.
At the start of any data quality process, you should also ensure your data gathering, storage, access, and privacy methods are compliant with national, market and other regulatory requirements.
The essence of quality data
Ensuring high-quality data involves proactive quality assurance, quality control interventions, and monitoring data KPIs like accuracy, completeness, and timeliness. Data quality challenges, such as achieving accuracy and consistency with data from diverse sources, directly impact the dependability of analytics.
Investing in data quality improvement measures can save money and greatly boost the efficiency of business operations. Undeniably, high-quality data is essential for businesses to make informed decisions, ensuring precision and reliability in the insights obtained from data analytics.
Transforming raw data into actionable insights
Transforming raw data into clear, actionable insights takes multiple steps, including:
- Data collection
- Data cleansing
- Data analysis
- Data visualization
By applying advanced analytics techniques and using specialized tools, we can analyze data to identify patterns, trends, and correlations, extracting insightful information.
Most business applications such as ERP, CRM and retail systems offer a growing range of analytic and AI-powered features. This democratizes the science of data analytics but doing it well can give smarter businesses the edge over those taking a less-focused approach.
The interplay between data volume and analytics precision
The relationship between data volume and analytics precision is a delicate balance. Larger datasets offer more opportunities for insights but also introduce greater complexity and the need for more advanced analytics tools and techniques. The immense volume of data that characterizes big data can be overwhelming for traditional storage and processing systems, challenging analytical precision.
As data volumes expand, data governance becomes essential to ensure responsible and ethical management of data across company departments. Improperly managed data quality in large volumes can lead to errors, making analytical outcomes less reliable and precise.
Navigating the data analytics process
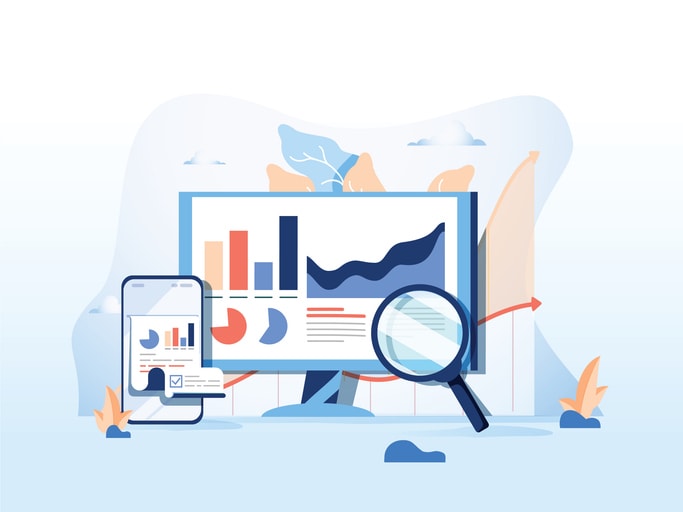
Navigating the data analytics process is challenging for smaller businesses with dedicated data scientists, but it’s a journey that starts with simple steps. The collection of the right data and processing it make sense of the information.
Throughout a first analytics trial or project, teams can use Kumospace to build and share knowledge, collaborate across each step, and overcome the inevitable queries that will arise. Kumospace provides immediate team situational awareness, showing who is available which supports teamwork, or showing when individuals are focused on a task. With a powerful interface to show and share applications and documents, Kumospace supports teams developing data analytics at speed, whatever the size or type of business,
These crucial steps in the analytics process provide the foundation for data analysis and insights:
- Collect meaningful data
- Process it using recognized techniques
- Analyze the data using historical or forward-looking references
- Draw insights from the data
Data collection can be classified into two main categories: primary and secondary, each with its own merits and challenges. One of the challenges in both categories is handling unstructured data, which requires specific techniques and tools for proper analysis.
Data collection: The starting point
The first step, data collection, involves:
- Gathering and evaluating information from multiple sources to find answers to research problems, forecast trends, and evaluate outcomes
- Collecting data directly from your own applications, customers, site visitors, and third parties, or even purchasing data to support analytics efforts
- The data collected serves as the starting point and is crucial for any subsequent analysis, decision-making, and visualization work within analytics processes.
Methods like surveys, interviews, observations, experiments, and focus groups are used for primary data collection.
For secondary data collection, analysts utilize sources such as:
- published materials
- online databases
- government and institutional records
- previously conducted research studies
Regardless of the method or source, data collection underpins the entire analytics process by providing the foundational base from which all further analysis, insights, and data-driven decisions stem.
Data processing: Making sense of information
The second step in the data analytics process, data processing, involves:
- Transforming raw data into a more readable format, often from production systems or extracting it from databases or spreadsheets
- Organizing the data to provide the context needed for computer interpretation and employee utilization
- Pre-processing the data to ensure it is valid, error-free and consistent
- Data input to the analytics tool
- Data output/interpretation stages that enable the translation of clean data to a system-understandable language
- Making the data results accessible in user-friendly formats such as graphs and documents.
The final stage is processed data storage where data is archived for future use and to comply with data protection laws, serving as a reference point for subsequent analysis. However, managing and processing substantial data volumes demands specialized infrastructure and tools, implying significant business costs.
Data analysis: Unveiling patterns and trends
The final step, data analysis, involves unveiling patterns and trends in the data. Examination of data through methods like:
- Regression to identify the dependent variables associated with a change
- Cluster analysis facilitates the identification of important patterns and trends.
- Time series analysis to find similarities in data
- Sentiment analysis to discover trends and cycles in time-related datasets
- With open text data, sentiment analysis is also used to interpret and classify positive and negative emotions, contributing to a deeper understanding of customer service opinions.
Visualization skills are key in data interpretation, they enable analysts to discern patterns and trends within the data. Techniques include:
- A/B testing is employed to compare different strategies and determine the most effective ones in a data-driven manner.
- Anomaly detection is integrated into data analysis to spot outliers that may indicate important deviations or new trends.
- KPIs are instrumental in revealing trends, patterns, and anomalies that suggest potential areas for business expansion or improvement.
These techniques, including statistical analysis, are essential for effective data analysis to improve decision-making, utilizing various data analytics techniques to achieve the best results.
Leveraging predictive analytics for future planning
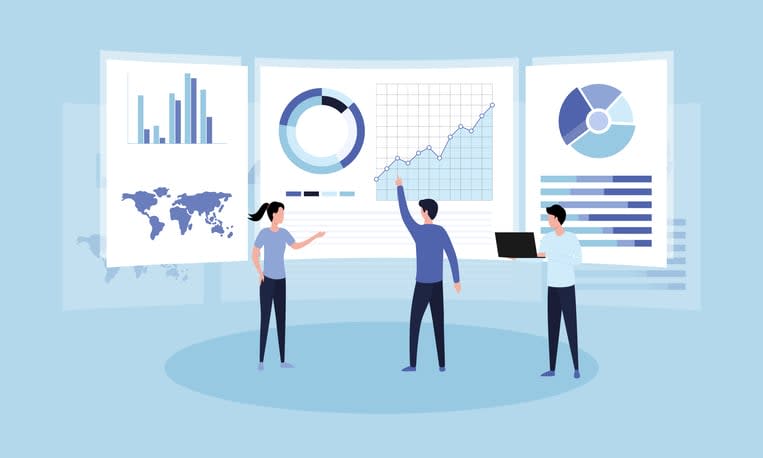
Predictive analytics is like a virtual crystal ball, providing foresight into the future—depending on the quality of your data. It uses historical data, statistical modeling techniques, and machine learning algorithms to make forecasts about future outcomes.
Time series analysis, a crucial component of predictive analytics, is used extensively for forecasting, trend analysis, and understanding temporal patterns in data collected over time.
The shift from intuition-based to data-driven strategies in decision-making through software, AI and IT advancements, underpin the role of predictive analytics. Predictive analytics bolsters decision-making capabilities across various domains by:
- Identifying patterns in the data
- Forecasting trends and answering ‘what if…’ questions
- Improving budget and resource allocation
- Helping leaders improve their strategic planning
The absence of predictive analytics in decision-making processes exposes organizations to competitive risks and can lead to missed opportunities.
Predictive modeling: anticipating customer behavior
Predictive behavior modeling helps support sound economic business decision-making and customers’ interests. It forecasts customer behavior through mathematical and statistical techniques, such as logistic regression, applied to historical and transactional data. Sales forecasting employs methods like linear regression and machine learning algorithms, including gradient boosting and random forests, which are adept at discerning varied data relationships and trends.
Customers are dynamically segmented into micro-segments through predictive modeling, based on their behaviors and demographic characteristics, with segments evolving as new data emerges. Advanced models identify each customer’s ‘segment route history,’ recognizing that behavior patterns change over time, to refine predictions of future behavior and value. The deployment of predictive analytics allows for more personalized recommendations, as seen with Spotify’s ‘Discover Weekly’ playlists generated based on individual user preferences and habits, enhancing user engagement and retention.
Sales forecasting: driving revenue with data
Predictive analytics in sales forecasting is like a roadmap to revenue growth. It analyzes historical data to predict future sales performance and develop revenue-generating strategies. The benefits of predictive sales forecasting include higher accuracy in predictions and the capacity to generate real-time forecasts that rapidly respond to changes in market conditions.
However, implementing predictive sales forecasting comes with many challenges, such as the need for accurate and comprehensive data collection, and ensuring sales teams adopt these strategies through training and support, boosting their effectiveness. With the speed of change in artificial intelligence and machine learning improving the precision of predictive sales forecasting significantly, its value increases in strategic sales planning and other areas.
Tools of the trade: Essential data analytics tools
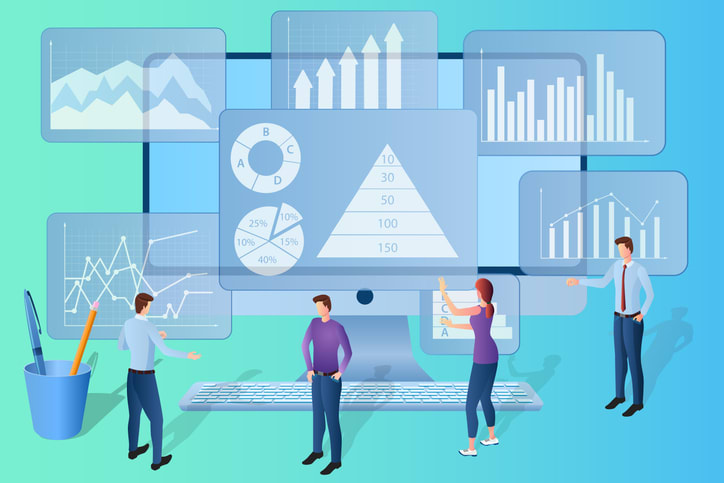
Just as an artist needs a brush to paint a masterpiece, data analysts need the right tools to extract valuable insights from data. Key technologies used in data analytics include data mining, text analytics, data visualization, and business intelligence. Tools and strategies for managing data volume include distributed computing, data compression, and effective data governance policies.
The future of data processing is trending towards cloud technology, offering faster processing, high-quality data, and valuable insights that benefit corporations and small companies. Data quality and data integrity are ensured through tools that focus on the usefulness, accuracy, and security of the data in the analysis process. Tools like SAS and Apache Spark assist with data mining and processing large sets aka big data, both integral to data analysis.
Data visualization: Bringing data to life
Data visualization is a critical component in data science that transforms large volumes of complex raw data into clear, actionable insights for strategic decision-making.
Data visualization is the art of presenting complex data in an easily interpretable format. It allows the audience to engage with and interpret the data through well-designed graphs and visualizations. During the COVID-19 pandemic, data visualization played a key role by making critical information understandable to society and aiding in explaining crucial decision-making processes to politicians.
Some key points about data visualization are:
- It helps present complex data in an easily interpretable format.
- Well-designed graphs and visualizations engage the audience and aid in data interpretation.
- Maintaining transparency and integrity is important in data visualization to avoid misleading representations.
Selecting the appropriate type of visualization is essential to accurately depict complex data and to uncover trends or patterns that inform strategic decisions. Some tools that can help with this are:
- Tableau: facilitates interactive chart creation and data exploration
- Klipfolio: allows the creation of custom dashboards from diverse services
- Looker: provides extensive visualization choices through its marketplace
- Zoho Analytics: integrated within the Zoho suite for robust reporting capabilities
Machine learning: automating analytical processes
Machine learning is the driving force behind many automated analytical processes. It significantly impacts data analytics by requiring high-quality data to train algorithms for accurate predictions and outcomes. The preparatory phase of machine learning in analytics entails processing the data using specialized algorithms tailored to the data’s source and purpose.
The versatility of tools like Qlik Sense, which incorporates AI, allows for quick calculations and access to numerous data sources, extending the scalability and interactivity offered by standard data visualization tools. Innovations such as multimodal AI and agentic AI show a trend toward systems that can process varied data inputs, exhibit autonomy, and perform goal-directed actions independently. The rise of open-source AI and the development of retrieval-augmented generation and customized AI models underscore the progress in creating more tailored and accurate AI-driven solutions.
The strategic value of data-driven decision-making
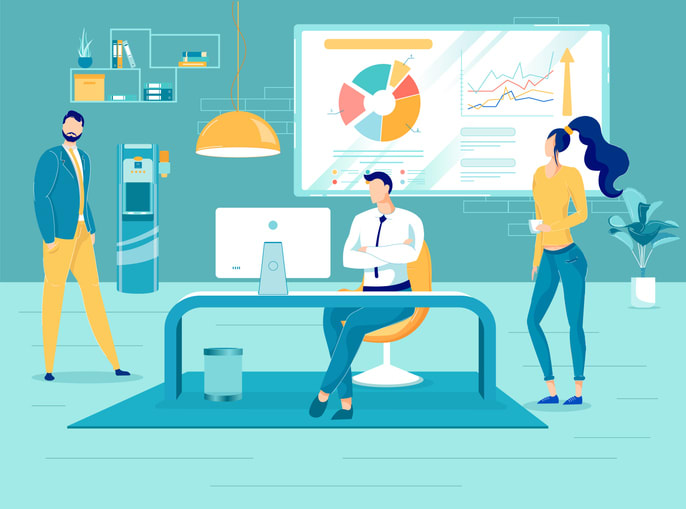
Data-driven decision-making is like a compass guiding businesses to success. It ensures the efficient utilization of resources, optimizing operational performance and reducing wastage. Investing in data and analytics can yield a six percent average increase in profits, which can rise to nine percent for investments sustained over five years.
The growing attention to AI ethics and security risks underscores the need for transparent and fair AI systems, emphasizing the strategic value of robust AI governance. Real-world application of data-driven decision-making is evident as organizations use survey-based research to inform planning processes, particularly in enhancing employee engagement strategies.
Boosting operational efficiency through analytics
Data analytics works like a detective, uncovering areas of inefficiency in operations or likely best steps to capture future market wins. Examples of how different industries use data analytics include:
- Manufacturing: Data analytics is crucial for predictive maintenance and real-time monitoring, which increases productivity and reduces costs.
- Retail: Data analytics helps teams manage inventory, optimize availability, and reduce waste.
- Healthcare: Data analytics can help manage staffing, ensuring the right resources are allocated at the right time.
- Transportation: Data analytics is used to optimize routes, reduce fuel consumption, and improve delivery times.
- Finance: Data analytics helps assess credit risk, improve operational efficiency and reduce defaults.
Across most businesses, operational efficiency KPIs, driven by analytics, highlight bottlenecks and underperformance, guiding businesses toward more streamlined processes. By anticipating maintenance needs and mitigating potential outages through analytics, organizations can maintain optimal performance levels, preventing costly downtime. Data analytics supports the identification of inefficiencies in existing workflows, enabling organizations to devise streamlined and cost-effective operations.
Data-driven identification of wasteful spending supports the strategic reallocation of resources, improving the overall financial health of an organization.
Informing marketing strategies with customer data
Customer data is like a goldmine of insights for marketers. Analyzing customer behavior and preferences through data analytics provides insights that enable more targeted and effective marketing strategies. Data-driven marketing enables:
- The segmentation of markets and definition of key customer types
- The creation of highly tailored marketing materials
- Targeting the right people at the right place and time improves the effectiveness of digital advertising.
Personalized marketing communications based on individual customer data creates a more relevant user experience, driving upsells and customer loyalty. E-commerce businesses optimize their marketing campaigns and personalize shopping experiences by understanding customer behavior through data analytics.
E-commerce platforms use data analytics to personalize product recommendations based on customers’ browsing and purchase histories, leading to increased sales and satisfaction. By leveraging KPIs, companies can optimize marketing and sales efforts, ensuring resources are allocated effectively and campaigns are adjusted for maximum ROI.
Retailers utilize data analytics for insights into customer trends, and preferences, and optimizing inventory management, which helps in forecasting demand and enhancing marketing efforts.
Measuring success: Key performance indicators in data analytics
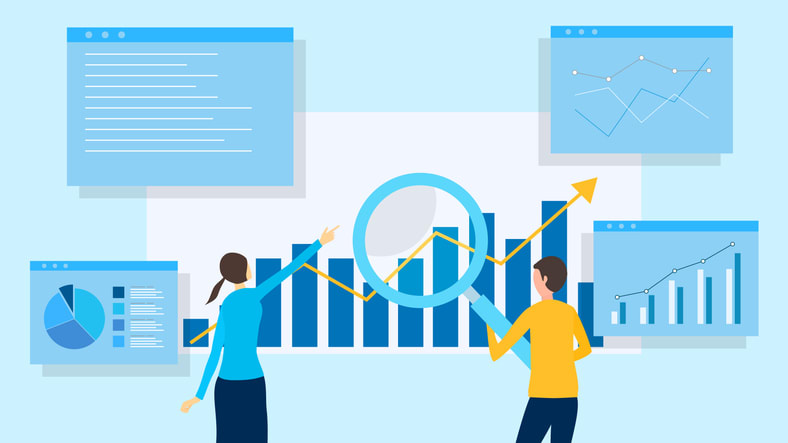
Key performance indicators (KPIs) guide data teams towards their business objectives. Well-defined KPIs are crucial to giving data analytics staff a clear direction, allowing for objective measurement of their impact.
KPIs ensure the work of data teams aligns with the strategic objectives of the organization, helping objectively evaluate their contributions towards these goals.
In marketing, tracking relevant KPIs is part of a data-driven strategy that leads to effective allocation of resources and optimization of marketing campaigns based on quantifiable performance metrics.
Setting benchmarks: Establishing relevant data points
Setting benchmarks is like drawing a map to success. Defining KPIs tailored to specific roles within the data team, such as analysts, engineers, and scientists, ensures measurable contributions toward business objectives. Setting benchmarks for KPIs requires the establishment of clear, strategic objectives to guide the measurement of specific performance aspects.
KPIs must be industry-relevant, customer-focused, and measurable based on reliable, accurate data. Prioritizing KPIs using impact evaluation and data feasibility matrices assures alignment with business goals and facilitates the adaption to business and market changes.
Continuous improvement: Using data to refine business practices
Continuous improvement is like honing a skill, the more you practice, the better you become. Continuous analysis of KPIs is key to defining success and pinpointing improvement opportunities within business processes. Tracking KPIs enables organizations to measure the return on investment of their data projects, ensuring fiscal responsibility in their data-driven initiatives.
Regular KPI discussions across the company nurture an environment of accountability and alignment on common goals for ongoing improvement. Data analysis catalyzes continuous improvement by allowing businesses to monitor performance and identify processes that can be improved.
Real-world impact: Case studies of data analytics in action
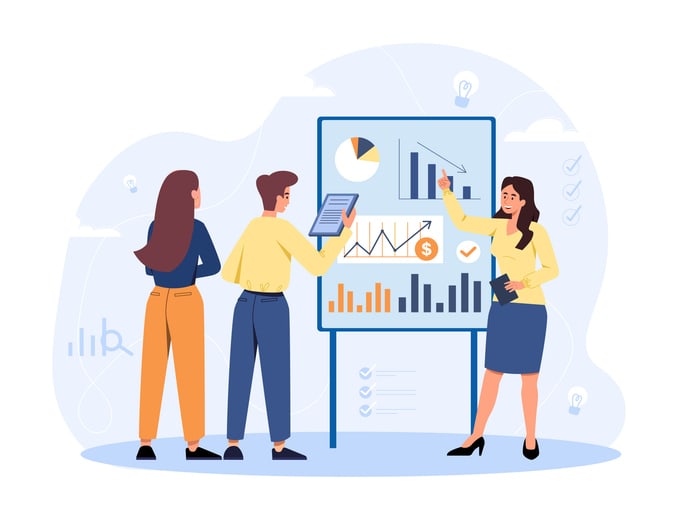
Real-world case studies are like a window into the tangible impact of data analytics. Companies in the energy management sector use data analytics for:
- Smart-grid management
- Optimizing energy consumption
- Energy distribution
- Building automation for utility services
Through the use of data analytics and business analytics, these companies can significantly enhance operational efficiency and decision-making processes.
Optimizing team performance with user engagement data
The case of Kumospace, a pioneer in virtual collaboration and business operations, underscores the critical role of data analysis in optimizing team performance and decision-making. By providing insights into user engagement and interaction patterns, Kumospace leverages data analytics to drive business success and strategic development.
Strategic development is driven by data insights
In another case, Biomat leveraged data analytics for strategic development. By creating an ETL solution to amalgamate data from multiple sources, Biomat was able to identify and rectify production bottlenecks, showcasing the transformative power of data insights in boosting business operations.
Summary
Data analytics, the compass of the digital age, guides businesses toward strategic decisions, operational efficiency, and customer satisfaction. By transforming raw data into actionable insights, businesses can navigate the complex landscape of the digital economy, harnessing the power of data to drive success. Whether it’s optimizing team performance, refining business practices, or driving strategic development, data analytics is the key to unlocking the potential of businesses in the digital age.
Frequently Asked Questions
Data is the foundation of all reporting in business, as it consists of plain facts and statistics collected during business operations, and is crucial for measuring and recording various activities.
Data and data analysis are crucial in real life as they help businesses identify trends, forecast future performance, understand customer behavior, manage risks, enhance operations, and direct resources effectively. These processes provide valuable insights across various industries, giving businesses a competitive edge and enabling them to make informed decisions.
In data analytics, data refers to raw information analyzed to derive insights and conclusions. This process encompasses a variety of techniques and technologies aimed at uncovering trends and solving problems through data analysis, leading to improved decision-making and business growth.
Data analytics tools tend to be technical, so teams need to rely on each other’s insights and strengths, building a knowledge base that all can access. Kumospace provides the powerful collaboration tools like whiteboards and ad hoc meetings to make this process immediate and easier, whatever analytics tools are in use.
Predictive analytics contributes to future planning by using historical data, statistical modeling, and machine learning to forecast future outcomes, improving decision-making and strategic planning.
KPIs play a crucial role in data analytics by providing clear direction for data teams and ensuring their work aligns with the strategic objectives of the organization. This allows for objective measurement of the impact on business objectives.